- Home
- Assessments
- Bioregional Assessment Program
- Hunter subregion
- Surface water numerical modelling for the Hunter subregion
- Model development
- Choice of seasonal scaling factors for climate trend
In developing a future series of climate input, the objective is to choose the set of global climate model (GCM) seasonal scaling factors that give the median change in mean annual precipitation in the Hunter subregion. There are 15 available GCMs (as presented in Table 3) with seasonal scaling factors for each of the four seasons: summer (December–February), autumn (March–May), winter (June–August) and spring (September–November).
For each GCM the change in mean seasonal precipitation that is associated with a 1 °C global warming is calculated. These seasonal changes are then summed to give a change in mean annual precipitation. The resulting changes in mean annual precipitation for a 1 °C global warming in the Hunter subregion are shown in Table 3 for each GCM. The 15 GCMs predict changes in mean annual precipitation ranging from –6.2% (i.e. a reduction in mean annual precipitation) to 8.3% (i.e. an increase in mean annual precipitation). The GCM with the median change is MRI (from the Meteorological Research Institute, Japan). The corresponding projected change in mean annual precipitation per degree of global warming is a reduction of 1.5%, or about 11 mm. The seasonal scaling factors for MRI are +5.4%, –3.4%, –8.1% and –1.8% for summer, autumn, winter and spring, respectively. In other words, projected increases in precipitation in the wettest season, summer, are offset by projected decreases in the other three seasons.
Table 3 List of 15 global climate models (GCMs) and their predicted change in mean annual precipitation across the Hunter subregion per degree of global warming
GCM |
Modelling group and country |
Change in mean annual precipitation (%) |
---|---|---|
CCCMA T47 |
Canadian Climate Centre, Canada |
8.3% |
MIUB |
Meteorological Institute of the University of Bonn, Germany Meteorological Research Institute of the Korea Meteorological Administration, Korea |
4.9% |
MIROC3 |
Centre for Climate Research, Japan |
3.7% |
CCCMA T63 |
Canadian Climate Centre, Canada |
2.7% |
NCAR-PCM |
National Center for Atmospheric Research, USA |
2.1% |
NCAR-CCSM |
National Center for Atmospheric Research, USA |
1.4% |
INMCM |
Institute of Numerical Mathematics, Russia |
0.7% |
MRI |
Meteorological Research Institute, Japan |
–1.5% |
CSIRO-MK3.0 |
CSIRO, Australia |
–1.6% |
GFDL2.0 |
Geophysical Fluid, Dynamics Lab, USA |
–1.7% |
IAP |
LASG/Institute of Atmospheric Physics, China |
–2.3% |
MPI-ECHAM5 |
Max Planck Institute for Meteorology, German Climate Computation Centre (DKRZ), Germany |
–3.1% |
IPSL |
Institut Pierre Simon Laplace, France |
–4.0% |
GISS-AOM |
NASA/Goddard Institute for Space Studies, USA |
–5.0% |
CNRM |
Meteo-France, France |
–6.2% |
Data: CSIRO (Dataset 7)
The seasonal scaling factors associated with MRI are used to generate trended precipitation inputs for the years 2013 to 2102. The trends assume global warming of 1 °C for the period 2013 to 2042, compared to 1983 to 2012. The global warming for 2043 to 2072 is assumed to be 1.5 °C and the corresponding scaling factors for this period are therefore multiplied by 1.5. The global warming for 2073 to 2102 is assumed to be 2 °C.
The scaling factors are applied to scale the daily precipitation in the climate input series that is generated for 2013 to 2102. The resulting annual precipitation time series for the Hunter subregion is shown in Figure 6. It depicts a cycle of 1983 to 2012 climate that is repeated a further three times but with increasingly trended climate change scalars. It can be seen from Figure 6 that the decrease in precipitation from 2013 to 2102 is less than the typical inter-annual variability. Furthermore, it reduces annual precipitation rates to levels that remain much higher than were typically encountered in the first half of the 20th century.
The blue line shows the time series. The red line is a centrally weighted moving average.
Data: Bioregional Assessment Programme (Dataset 8)
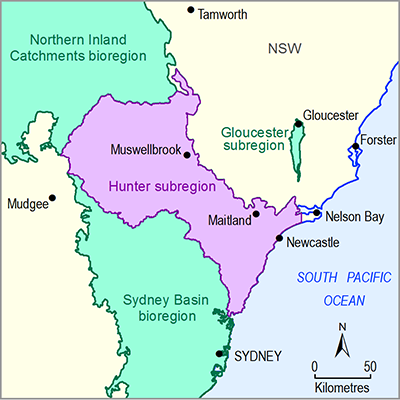