2.7.5.4.1 Pilliga riverine (upland and lowland)
Table 39 summarises the elicitation design matrix for the projected foliage cover of riparian trees in the riverine landscape classes in the Pilliga region. The first three design points – design point identifiers 1 to 3 – address the predicted variability (across the streams in the landscape class during the reference interval) in the zero-flow regime. Note that the design point identifiers are simply index variables that identify the row of the elicitation design matrix. They are included here to maintain an auditable path between analysis and reporting.
The first design points provide for an estimate of the uncertainty in mean projected foliage cover across the landscape class in the reference year 2012 (Yref). The remaining design points represent hydrological scenarios that span the uncertainty in the values of the hydrological response variables in the relevant time period of hydrological history associated with the short- (2042) and long- (2102) assessment years.
Table 39 Elicitation design matrix for the annual mean projected foliage cover of riparian trees along Pilliga riverine landscape classes that includes: yellow box, white cypress pine, Eucalyptus crebra, dirty gum, Blakely's red gum, Angophora floribunda, Eucalyptus fibrosa, fuzzy box in the Namoi subregion zone of potential hydrological change
Receptor impact modelling elicitation design matrix for annual mean projected foliage cover in a transect of 50 m length and 20 m width that extends from first bench (‘toe’) on both sides of the stream in permanent and temporary upland streams. Design points for Yref in the future (short- and long-assessment periods) are calculated during the receptor impact modelling elicitation workshop using elicited values for the receptor impact variable in the reference period. All other design points (with identifiers Id) are either default values or values determined by groundwater and surface water modelling. Hydrological response variables are as described in Table 37. na = not applicable
Data: Bioregional Assessment Programme (Dataset 4)
Design point identifiers 15 through to 108 (as listed in Table 39) represent combinations of the three hydrological response variables (dmaxRef, tmaxRef and ZQD), together with high and low values of Yref (see companion submethodology M08 (as listed in Table 1) for receptor impact modelling (Hosack et al., 2018)). The high and low values for Yref were calculated during the receptor impact modelling workshop following the experts’ response to the first design point, and then automatically included within the design for the elicitations at the subsequent design points.
The receptor impact modelling methodology allows for a very flexible class of statistical models to be fitted to the values of the receptor impact variables elicited from the experts at each of the design points (companion submethodology M08 (as listed in Table 1) for receptor impact modelling (Hosack et al., 2018)). The model fitted to the elicited values of mean foliage cover for the Pilliga riverine landscape classes is summarised in Figure 29 and Table 40. The fitted model takes the form:
(7) |
where is an intercept term (a vector of ones),
is a binary indicator variable scored 1 for the case of an assessment in the short- or long-assessment year,
is a binary indicator variable scored 1 for the case of an assessment in the long-assessment year,
is a continuous variable that represent the value of the receptor impact variable in the reference year (Yref, set to zero for the case of an assessment in the reference year), and
are the (continuous or integer) values of the three hydrological response variables (dmaxRef, tmaxRef and ZQD). Note that the modelling framework provides for more complex models, including quadratic value of, and in interactions between, the hydrological response variables but in this instance the simple linear model (Equation 7) was identified as the most parsimonious representation of the experts’ responses.
The model estimation procedure adopts a Bayesian approach. The model coefficients ( are assumed to follow a multivariate normal distribution. The Bayesian estimation procedure quantifies how compatible different values of the parameters of this distribution are with the data (the elicited expert opinion) under the model. The (marginal) mean and 80% central credible intervals[3] of the three hydrological response variable coefficients are summarised in partial regression plots in Figure 29, while Table 40 summarises the same information for all seven model coefficients.
The model indicates that the experts’ opinion provides strong evidence for Yref having a positive effect on average percent projected foliage cover. This suggests that given a set of hydrological response variable values in the future, a site with a higher foliage cover at the 2012 reference point is more likely to have a higher foliage cover in the future than a site with a lower foliage cover value at this time point. This reflects the lag in the response of foliage cover to changes in hydrological response variables that would be expected of mature trees with long life spans.
The model also indicates that the experts’ opinion provides some evidence for dmaxRef having a negative effect on average percent projected foliage cover (Figure 29). This suggests that percent projected foliage cover will decrease as groundwater drawdown increases due to coal resource development. The model predicts that (holding all other hydrological response variables constant at the mid-point of their elicitation range) the mean of the average percent projected foliage cover will drop from just under 12% without any change in groundwater level, to about 10% if the levels decrease by 50 m relative to the reference level in 2012. There is, however, considerable uncertainty in these predictions, with an 80% chance that the foliage cover will lie somewhere between approximately 8% and 16% on the short-assessment period, and somewhere between roughly 7% and 17% in the long-assessment period, with a 6 m drop in groundwater level. In relation to dmaxRef, Yrs2tmax is also found slightly significant, but with a positive effect. This suggests that a maximum drawdown happening very early would have a larger effect on foliage cover than a maximum drawdown reached after a long time.
The model indicates that the experts’ opinion provides strong evidence for ZQD (zero-flow days (averaged over 30 years) subsequently referred to in this Section as ‘zero-flow days’) having a negative effect on average percent projected foliage cover. The model predicts that (holding all other hydrological response variables constant at the mid-point of their elicitation range) the mean of the average percent projected foliage cover will decrease from just under 25% without any zero-flow days to about 10% if the number of zero-flow days increases to 180.
Finally, the model also indicates some diverging influence between short-term and long-term influence (holding hydrological response variables constant). For the short-assessment period, experts believe in a relative increase of foliage cover, while they are left uncertain about the long‑term assessment effect. An interpretation is that the effects of changes in hydrology are not immediate on foliage cover, and 2102 is a very long time into the future to make assessments without uncertainty.
Dashed vertical lines show hydrological response variable range used in the elicitation. Hydrological response variables ZQD and dmaxRef are as defined in Table 37. Yrs2tmaxRef is the difference between tmaxRef and the assessment year that is relevant for the prediction (2012, 2042 or 2102). The numbers on the y-axis range from 0 to 1 as the receptor impact model was constructed using the proportion for the statistical modelling. They should be interpreted as a percent foliage cover ranging from 0 to 100%.
Data: Bioregional Assessment Programme (Dataset 4)
Table 40 Mean, 10th and 90th percentile of the coefficients of the receptor impact model for annual mean projected foliage cover along Pilliga riverine landscape classes
Future1 is the indicator variable for the short-assessment year (2042). Long1 is the indicator variable for the long-assessment year (2102). Yref quantifies the value of the receptor impact variable during the reference period. Hydrological response variables dmaxRef and ZQD are as defined in Table 37. Yrs2tmaxRef is the difference between tmaxRef and the assessment year that is relevant for the prediction (2012, 2042 or 2102).
Data: Bioregional Assessment Programme (Dataset 4)
Table 41 summarises the elicitation matrix for the average number of families of aquatic macroinvertebrates in instream pool habitats. The first six design points – design points 1 to 7 as shown – address the predicted variability (across the landscape class in the reference interval) in ZQD and ZME, capturing the lowest and highest predicted values together with two intermediate values. These design points provide for an estimate of the uncertainty in aquatic macroinvertebrate family abundance across the landscape class in the reference year 2012 (Yref).
Design points 21 to 213 inclusive (as listed in Table 41) represent scenarios that span the uncertainty in the predicted values of ZQD and ZME in the relevant time period of hydrological history associated with the short- (2042) and long- (2102) assessment years, combined with high and low values of Yref, as well as groundwater drawdown and time to drawdown combinations. Again, the high and low values for Yref were calculated during the receptor impact modelling workshop.
The fitted model for number of families of macroinvertebrates in instream pool habitats takes the form:
|
where is an intercept term (a vector of ones),
is a binary indicator variable scored 1 for the case of an assessment in the short- or long-assessment year,
is a binary indicator variable scored 1 for the case of an assessment in the long-assessment year,
is a continuous variable that represent the value of the receptor impact variable in the reference year (Yref, set to zero for the case of an assessment in the reference year), and
are the (continuous or integer) values of the four hydrological response variables (dmax, tmax, ZQD and ZME). Note that the modelling framework provides for more complex models, including quadratic value of, and in interactions between, the hydrological response variables but in this instance the simple linear model (Equation 8) was identified as the most parsimonious representation of the experts’ responses.
Table 41 Elicitation design matrix of the average number of families of aquatic macroinvertebrates in instream pool habitat along Pilliga riverine landscape classes in Namoi subregion zone of potential hydrological change
Receptor impact modelling elicitation design matrix for average number of families of aquatic macroinvertebrates in instream pool habitat in permanent and temporary upland and lowland streams (Pilliga riverine landscape classes). Design points for Yref in the future (short- and long-assessment periods) are calculated during the receptor impact modelling elicitation workshop using elicited values for the receptor impact variable in the reference period. All other design points (with identifiers Id) are either default values or values determined by groundwater and surface water modelling. Hydrological response variables are as described in Table 37.
na = not applicable
Data: Bioregional Assessment Programme (Dataset 4)
The hydrological response variable in the macroinvertebrate model varies during the reference interval and the future interval. The model indicates that the experts’ elicited information supports the hypothesis that an increase in ZQD and/or ZME will have a slightly negative effect on the number of families of macroinvertebrates despite the experts being somewhat uncertain about its average value. The model suggests that it can vary across the landscape class from less than 15 to almost 20 under conditions of constant flow (ZQD = zero), holding all other covariates at their mid-values. As the number of zero-flow days increases, however, experts were of the opinion that the number of families would drop quite dramatically with values between 13 and 6 (under very intermittent flow conditions ZQD >150 days) (Figure 30).
There was very little evidence in the elicited data to suggest that this effect would be substantially different in the future assessment years. Again, this is indicated by the almost identical partial regression plots in the reference, short- and long-assessment years (Figure 30), and the relatively large negative 10th and positive 90th percentiles for the long and future coefficients in Table 42. The model does, however, suggest that the experts’ uncertainty increased a lot for predictions in the future assessment years relative to the reference year.
The best-fitting model is unable to eliminate the possibility that the average number of families of macroinvertebrates in the reference years has no influence on its number in the future years. This is indicated by the fact the model automatically dropped this variable from the model. This suggestion is consistent with the hypothesis that there is likely to be very little lag in the response of this short-lived species to changes in the hydrological response variables.
The model also indicates that the experts’ opinion provides strong evidence for dmaxRef having a negative effect on macroinvertebrates in instream pool habitats. This suggests that the number of families of macroinvertebrates will decrease as groundwater drawdown increases due to coal resource development. The model predicts that (holding all other hydrological response variables constant at the mid-point of their elicitation range) the number of families will drop from just under 12 without any change in groundwater level, to about 6 if the levels decrease by 55 m relative to the reference level in 2012. There is, however, considerable uncertainty in these predictions, with an 80% chance that the number of families will lie somewhere between approximately 1 and 12 on the short-assessment period and in the long-assessment period. In relation to the time of drawdown, Yrs2tmax is not found significant, meaning that the time at which the maximum drawdown will occur does not carry much influence over the number of families of aquatic macroinvertebrates.
Dashed vertical lines show hydrological response variable range used in the elicitation. Hydrological response variables are as defined in Table 37. Yrs2tmaxRef is the difference between tmaxRef and the assessment year that is relevant for the prediction (2012, 2042 or 2102).
Data: Bioregional Assessment Programme (Dataset 4)
Table 42 Mean, 10th and 90th percentile of the coefficients of the receptor impact model for average number of families of aquatic macroinvertebrates in instream pool habitat in Pilliga riverine landscape classes
Future1 is the indicator variable for the short-assessment year (2042). Long1 is the indicator variable for the long-assessment year (2102). Hydrological response variables are as defined in Table 37. Yrs2tmaxRef is the difference between tmaxRef and the assessment year that is relevant for the prediction (2012, 2042 or 2102).
Data: Bioregional Assessment Programme (Dataset 4)
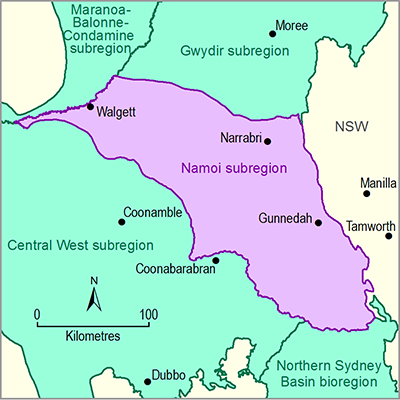
Product Finalisation date
- 2.7.1 Methods
- 2.7.2 Prioritising landscape classes for receptor impact modelling
- 2.7.3 'Floodplain or lowland riverine' landscape group
- 2.7.4 'Non-floodplain or upland riverine' landscape group
- 2.7.5 Pilliga riverine landscape classes
- 2.7.6 'Rainforest' landscape group
- 2.7.7 'Springs' landscape group
- 2.7.8 Limitations and gaps
- Citation
- Acknowledgements
- Contributors to the Technical Programme
- About this technical product