- Home
- Assessments
- Bioregional Assessment Program
- Hunter subregion
- 2.6.2 Groundwater numerical modelling for the Hunter subregion
- 2.6.2.1 Methods
- 2.6.2.1.2 Groundwater numerical modelling
In the Hunter subregion, the groundwater model has been developed in the Multiphysics Object-Oriented Simulation Environment (MOOSE) (Section 2.6.2.3). To be fit for the purposes of a bioregional assessment (BA), the groundwater model needs to satisfy the criteria listed in Table 3. The remainder of this section discusses each of these criteria having regard to the numerical modelling approach undertaken in the Hunter subregion.
Table 3 Assessment of groundwater numerical modelling approach in the Hunter subregion
2.6.2.1.2.1 Prediction of hydrological response variables
The objective of the numerical modelling in BAs is to assess hydrological changes arising from coal resource development using a probabilistic approach. In the Hunter subregion, the CRDP includes existing open-cut and underground mining operations, proposals to expand existing open-cut and underground mines and proposals for new open-cut and underground mines (see Section 2.3.4 of companion product 2.3 for the Hunter subregion (Dawes et al., 2018)). There are no CSG developments.
The groundwater and surface water models predict changes for a set of hydrological response variables, chosen to represent important hydrological characteristics of the system or landscape class (e.g. flow volumes, flow frequencies). Some of the hydrological outputs become inputs to receptor impact models through which the potential impacts of coal resource development on some water-dependent assets can be evaluated. Receptor impact models will not necessarily be generated for all water-dependent assets, so the modelled hydrological outputs will also be used to inform more qualitative assessments of other potentially impacted water-dependent assets.
The hydrological response variables for groundwater are (i) maximum difference in drawdown for one realisation within an ensemble of groundwater modelling runs results, obtained by choosing the maximum of the time series of differences between two futures (dmax) and (ii) time of maximum change (tmax). Drawdown is the difference in groundwater level between the baseline and CRDP within a regional-scale, unconfined aquifer that spans the entire model domain. These variables are generated in the model at the groundwater model nodes shown in Figure 3. Although change in baseflow is an output of the groundwater model, it is an input into the surface water modelling and therefore encapsulated within the set of surface water hydrological response variables (see companion submethodology M06 (as listed in Table 1) for surface water modelling (Viney, 2016)). The surface water – groundwater nodes in Figure 3 show where change in baseflows are generated in the groundwater model. Changes in the nine hydrological response variables for streamflow due to the additional coal resource development are reported in companion product 2.6.1 for the Hunter subregion (Zhang et al., 2018). Figure 3 shows the location of the surface water model nodes relative to the groundwater model nodes where surface water – groundwater fluxes are calculated.
The groundwater model is run many times using a wide range of parameter values to generate an ensemble of predictions. From this set of runs, a probability distribution is defined for each groundwater hydrological response variable at each groundwater model node in the subregion. This distribution summarises the uncertainty in the prediction (Section 2.6.2.8).
Figure 3 Groundwater and surface water model nodes in the Hunter subregion
ACRD = additional coal resource development, CRDP = coal resource development pathway
The maximum extent of the coal resource developments in CRDP is equal to the union of extents for baseline and ACRD.
Data: Bioregional Assessment Programme (Dataset 1, Dataset 2, Dataset 3, Dataset 4); CSIRO (Dataset 5)
Pumping water that flows from the coal seam, interburden and weathered rock into the working area during mining produces a cone of drawdown and a drop in the watertable around the worked area. Where drawdowns expand into alluvial aquifers that intersect the river channel, the flux of water from the alluvial aquifer to the river will tend to decrease. To represent this surface water – groundwater interaction, the groundwater model must either represent alluvial aquifers and the river network in its model structure or interface with an alluvial groundwater model that does. A surface water model constructed to represent the same river network can receive these changes in baseflow at specified points along its network to represent the combined effect of changes to surface runoff and groundwater input on streamflow. Since groundwater and surface water systems operate at different temporal scales, the models used to represent these processes run on different time steps. Streamflow is very responsive to individual rainfall events and is usually modelled at a daily time step or finer. Groundwater levels in shallow, unconsolidated alluvium are also responsive to changes in rainfall and river stage, but also to exchanges with deeper, intermediate and regional-scale groundwater aquifers in more consolidated material (i.e. lower transmissivities), which respond relatively slowly to changes in rainfall recharge. To predict these intermediate and regional-scale groundwater systems, a monthly or more infrequent time step can suffice.
While fully coupled surface water – groundwater model codes are available (e.g. HydroGeoSphere, Brunner and Simmons, 2012), their use is not feasible within BAs due to their high data requirements for parameterisation and operational constraints. The latter relates mainly to the general numerical instability of such models and long runtimes which would severely limit a probabilistic uncertainty analysis that requires the models to be evaluated hundreds of times with vastly different parameter sets.
For the Hunter subregion, the modelling suite includes the Australian Water Resource Assessment landscape (AWRA-L) water balance model (Viney et al., 2015) to calculate the surface runoff to streams; the MOOSE groundwater model to predict watertables and change in baseflows (detailed in this product); and the AWRA-R (river) model (Dutta et al., 2015) via which surface runoff and change in baseflow are propagated downstream. The individual models have different spatial and temporal resolution which requires a set of customised processing steps to upscale or downscale model data to allow the models to be linked.
Figure 4 illustrates the model sequencing, parameters exchanged between models and the outputs generated at model nodes to inform the receptor impact modelling. The MOOSE, AWRA-L and AWRA-R baseline runs predict the hydrological changes of coal mines that were commercially producing coal as at December 2012. The corresponding CRDP runs predict the combined hydrological changes of the baseline coal resource development (baseline) and those expected to begin commercial production after 2012 (see Section 2.3.4 of companion product 2.3 for the Hunter subregion (Dawes et al., 2018)).
The difference in predicted drawdown between baseline and CRDP runs, expressed in terms of dmax and tmax, yields the predicted hydrological changes due to the additional coal resource development in the Hunter subregion. In the receptor impact analysis, the ecological consequences of the predicted changes in HRVs in the fractured rock aquifers and alluvial aquifers are assessed.
Figure 4 Model sequence for the Hunter subregion
AWRA-L = rainfall-runoff model; AWRA-R = river model; CRDP = coal resource development pathway; dmax = maximum difference in drawdown for one realisation within an ensemble of groundwater modelling runs results, obtained by choosing the maximum of the time series of differences between two futures; GW = groundwater; ΔHRV = change in hydrological response variable; MOOSE = groundwater model; ΔQb = change in baseflow relative to no development baseflow; Qr = surface runoff; Qt = total streamflow; SW = surface water; tmax = year of maximum change
2.6.2.1.2.2 Design and construction
According to the Australian groundwater modelling guidelines (Barnett et al., 2012), the design and construction of a groundwater model should meet a clear set of objectives (see preceding section) and provide some measure of model confidence. The model confidence level is an a priori categorisation of a groundwater model to reflect its predictive capability and is a function of model complexity, prediction time frame and data availability. As explained in companion submethodology M07 (as listed in Table 1) for groundwater modelling (Crosbie et al., 2016), the groundwater models in the BAs are all Class 1 (lowest level) models because they are required to make predictions of unprecedented stresses over time frames longer than the periods with data available to constrain the model.
Further technical detail of the conceptualisation, parameterisation and implementation are provided in Section 2.6.2.3 for the MOOSE groundwater model and in companion product 2.6.1 for the Hunter subregion (Zhang et al., 2018) for the AWRA-L and AWRA-R models.
2.6.2.1.2.3 Integration with sensitivity and uncertainty analysis workflow
Companion submethodology M09 (as listed in Table 1) for propagating uncertainty through models (Peeters et al., 2016) discusses in detail the propagation of uncertainty through numerical models in the BAs. Figure 5 summarises the uncertainty propagation workflow which consists of four major steps:
- Design of experiment: large number of model chain evaluations with a wide range of parameter values
- Train emulators, i.e. statistical models that mimic the numerical model behaviour, for:
- each hydrological response variable at each model node
- objective function tailored to each hydrological response variable at each model node
- Create posterior parameter probability distribution through Approximate Bayesian Computation Markov chain Monte Carlo
- Sample the posterior parameter probability distribution to generate the posterior probability distribution for each hydrological response variable at each model node.
Figure 5 Uncertainty analysis workflow
ABC MCMC = Approximate Bayesian Computation Markov chain Monte Carlo; HRV = hydrological response variable
The first step is to identify the parameters of the model chain to include in the uncertainty analysis and to define a wide range that represents the plausible range of the parameters. A large number of model chain evaluations are carried out, sampling extensively from this parameter range. For each evaluation the corresponding predicted changes in hydrological response variables at the model nodes are stored, together with the predicted equivalents to the observations. The latter are summarised into objective functions, tailored to each hydrological response variable.
This information forms the basis for the subsequent uncertainty analysis. In the uncertainty analysis, the prior parameter distributions – the most likely range of the parameter values based on data and expert knowledge – are constrained with the available relevant data using the Approximate Bayesian Computation methodology. This results in a posterior parameter distribution, tailored to a specific hydrological response variable, which subsequently can be sampled to generate a probability distribution at each model node.
This type of uncertainty analysis requires a very large number of model evaluations. To reduce the computational load associated with running the numerical model, the original model chain in the uncertainty analysis is replaced by emulators, statistical functions that closely mimic the effect of parameter values on predictions. These emulators take little time to evaluate and are straightforward to integrate in the uncertainty analysis workflow.
To incorporate the model chain into the uncertainty analysis it needs to be scripted so the parameter values can be changed in an automated fashion, be evaluated from a command line on high performance computers and, most importantly, be numerically stable so that the model converges for a wide range of parameter values.
The three models in the model chain for the Hunter subregion have text files as input files and can be executed from the command line. The robustness of each model is tested through a stress test in which a selection of extreme parameter combinations is evaluated. While this does not guarantee that all model evaluations will converge, it provides confidence that the majority of parameter combinations will.
Section 2.6.2.7 and Section 2.6.2.8 provide details of the implementation of this uncertainty propagation workflow for the Hunter groundwater model. The uncertainty analysis for the AWRA-L model is in Section 2.6.1.5 and Section 2.6.1.6 of companion product 2.6.1 for the Hunter subregion (Zhang et al., 2018). These sections also have a qualitative uncertainty analysis that provides a structured discussion of the assumptions and model choices not included in the numerical uncertainty analysis and the perceived effect on the predictions.
2.6.2.1.2.4 Constraining regional results with local information
The main aim of a BA is to provide a regional-scale assessment of the potential hydrological changes and impacts that accumulate over time and space from multiple coal resource developments. Results from this assessment identify areas within the Hunter subregion that are potentially at risk from additional coal resource development. While some model parameters are locally constrained by observation data, the range of most parameters are informed by regional data.
The stochastic, prediction focussed approach permits the use of local scale information, such as contained in environmental assessments for the individual mines or from other local studies, to constrain the regional parameterisation, without the need to run the computationally intensive regional scale groundwater model again. In section 2.6.2.8.1.4, this concept is illustrated for the Wyong area.
2.6.2.1.2.5 Water balance components
A secondary objective of the numerical models is to inform the water balance reporting in companion product 2.5 for the Hunter subregion (Herron et al., 2018). The groundwater model and AWRA models produce estimates of the water balances under baseline and CRDP.
2.6.2.1.2.6 Transparent and reproducible model outputs
An overarching requirement of the BAs is for all model outputs to be transparent and reproducible.
Input data, model files (including the pre- and post-processing scripts and executables), and results are available at www.bioregionalassessments.gov.au.
As the evaluation of the model chain is a highly automated and scripted process, it is possible to reproduce the results reported in this product using the scripts and executables, provided the computational resources are available.
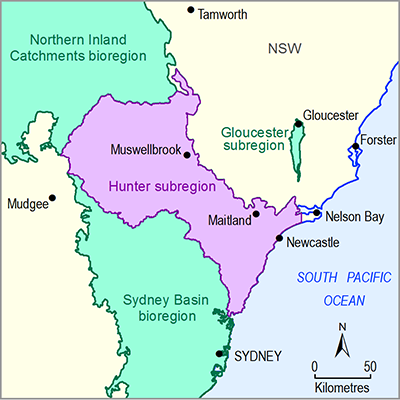
Product Finalisation date
- 2.6.2.1 Methods
- 2.6.2.2 Review of existing models
- 2.6.2.3 Model development
- 2.6.2.4 Boundary and initial conditions
- 2.6.2.5 Implementation of the coal resource development pathway
- 2.6.2.6 Parameterisation
- 2.6.2.7 Observations and predictions
- 2.6.2.8 Uncertainty analysis
- 2.6.2.9 Limitations and conclusions
- Citation
- Acknowledgements
- Currency of scientific results
- Contributors to the Technical Programme
- About this technical product