- Home
- Assessments
- Bioregional Assessment Program
- Gloucester subregion
- 2.7 Receptor impact modelling for the Gloucester subregion
- 2.7.3 'Riverine' landscape group
- 2.7.3.2 'Perennial – gravel/cobble streams' landscape class
2.7.3.2.1 Qualitative mathematical model
The qualitative model for the reaches of the ‘Perennial – gravel/cobble streams’ landscape class (Figure 17) focuses on the riparian-dependent community, the dynamics of which are strongly influenced by stream hydrology. The model recognises that woody riparian vegetation provides a critical role in stabilising stream banks, and in supplying large woody debris to the stream channel. This debris forms key habitat elements for multiple species, as well as providing allochthonous inputs of organic matter that in turn drives production of various macroinvertebrate populations (Boulton et al., 2014). Seedlings of woody vegetation species have greater survival in the presence of stable stream banks, which is facilitated by the binding of stream bank sediments by roots of trees. Herbaceous vegetation, which includes both terrestrial and aquatic plants, also provides inputs of organic matter.
The model identifies stuttering frogs (Mixophyes balbus) as a riparian species of particular interest. The egg laying and egg maturation of these frogs depends on spring baseflows, and the tadpole life stage depends on habitat elements provided by large woody debris (NSW Scientific Committee, 2002). The nationally endangered regent honeyeater is also included in the model due to its dependency on woody riparian vegetation (i.e. river sheoaks) for nesting habitat (Catterall et al., 2007; TSSC, 2015).
Stream macroinvertebrates in the model are classified into three groups based on their affinity for different aspects of stream velocity and flow, namely still-water macroinvertebrates, which occupy pool habitats, and slow- and high-flow macroinvertebrates, which are both associated with fast‑water habitats. The model includes two general groups of macroinvertebrate predators, differentiated by their mode of feeding. The benthic-feeding platypus consumes slow- and high‑flow benthic macroinvertebrates (NSW Office of Environment and Heritage, 2014), while still‑water macroinvertebrates are targeted by wading and diving birds (e.g. black bittern and kingfisher). The model also depicts fine sediment deposition as suppressing primary production and populations of the slow- and high-flow benthic macroinvertebrates. Recruitment of macroinvertebrate populations and woody riparian vegetation are both aided by delivery of propagules in flow from upstream reaches of the stream channel.
The model identifies three surface water flow regimes that regulate key physical and ecological processes in the riparian system (Figure 11). Overbench (and bank-full) flows, with return intervals of two to five times per year (flow regime 1), were described by the participants of the qualitative modelling workshop as being a key factor in primary production: the scouring flows they produce rejuvenate production of benthic algae and remove fine sediments from stream substrates (Brierley and Fryirs, 2005). These flows also provide a source of organic matter by transporting leaf-fall from riparian trees into the stream channel (Boulton et al., 2014). Overbench flows also increase survivorship of both adult and seedlings of woody riparian vegetation (Robson et al., 2009). The model identifies a second flow regime (flow regime 2) associated with overbank flows with a 2- to 5-year return interval. This regime is considered to be key in lateral transport of organic matter from the floodplain into the stream channel. Overbank flows also increase soil moisture of floodplain soils, which contributes to survivorship of woody riparian vegetation. Flow regimes 1 and 2 are depicted as having a positive influence on upstream recruitment.
The third flow regime (flow regime 3) describes the role of baseflow conditions being above very low or minimum levels, which is important for a variety of species, including stream macrophytes (herbaceous vegetation), high-flow macroinvertebrates, and the stuttering frog (for egg laying in spring), and for the survival of seedlings of woody riparian vegetation. As previously stated, cease-to-flow events can create chains of pools, isolated pools or completely dry riverbeds, depending on riverbed morphology (Robson et al., 2009). The flow regime also influences riffle habitats through changes in water quality and reduced habitat area, as riffles dry out and contract.
Accessible groundwater levels are identified in the model as being critical for survival of adult woody riparian vegetation. Finally, rainfall (precipitation) is identified as important for the survival of seedlings of woody riparian vegetation, particularly during periods of summer low flows.
Variables are: bank stability (BS), fine particulate organic matter (FPOM), fine sediments (FS), flow regimes (FR1, FR2 and FR3), groundwater (GW), high-flow macroinvertebrates (HF MI), herbaceous vegetation (includes aquatic macrophytes) (HV), large woody debris (LWD), platypus (Platy), primary production (PP), precipitation (Ppt), predators (Pred 1 and Pred 2), regent honeyeater (RHE), seedlings (Seedl), slow-flow macroinvertebrates (SF MI), stuttering frogs (SF), still-water macroinvertebrates (SW MI), upstream recruitment (Ur), wading and diving birds (W&DB), woody riparian vegetation (WRV).
Data: Bioregional Assessment Programme (Dataset 5)
Qualitative mathematical modelling enables bioregional assessments (BAs) to consider the potential for coal resource development to impact hydrological variables either individually or in a cumulative (combined) fashion (companion submethodology M10 (as listed in Table 1) for analysing impacts and risks (Henderson et al., 2018)). Surface water and groundwater modelling predict significant potential impacts of coal mining to the three flow regimes and groundwater. Considering all combinations of plausible impacts suggests that there are 15 types of potential (some cumulative) impact scenarios that may occur in this landscape class due to individual or combined changes in the hydrological regimes identified in the model (Table 7).
Table 7 Summary of the cumulative impact scenarios for the riparian-dependent community in the ‘Perennial – gravel/cobble streams’ landscape class for the Gloucester subregion
Cumulative impact scenarios are determined by combinations of no change (0) or a decrease (–) in the following signed digraph variables: flow regime 1 (FR1), flow regime 2 (FR2), flow regime 3 (FR3) and depth to groundwater (GW). Scenario C10 shows the changes to these variables under the coal resource development pathway (CRDP).
Data: Bioregional Assessment Programme (Dataset 5)
Qualitative analysis of the signed digraph model (Figure 17) under each of the impact scenarios predicts a consistently negative response across virtually all the variables within the riparian-dependent community (Table 8). While some variables have a response prediction of zero for many or all of the scenarios (i.e. herbaceous vegetation), and fine sediments has a positive response prediction, all other variables are predicted to decline in their abundance, level or intensity with a relatively high level of sign determinacy.
Table 8 Predicted response of the signed digraph variables of the riparian-dependent community in the ‘Perennial – gravel/cobble streams’ landscape class to changes (some cumulative) in hydrological response variables for the Gloucester subregion
Qualitative model predictions that are completely determined are shown without parentheses. Predictions that are ambiguous but with a high probability (0.80 or greater) of sign determinacy are shown with parentheses. Predictions with a low probability (less than 0.80) of sign determinacy are denoted by a question mark. Zero denotes a completely determined prediction of no change.
Data: Bioregional Assessment Programme (Dataset 5)
2.7.3.2.2 Temporal scope, hydrological response variables and receptor impact variables
In BAs, the potential ecological impacts of coal resource development are assessed in two future years – 2042 and 2102. These are labelled as the short- and long-assessment years, respectively. Potential ecological changes are quantified in BAs by predicting the state of a select number of receptor impact variables in the short- and long-assessment years. These predictions are made conditional on the values of certain groundwater and surface water statistics that summarise the outputs of numerical model predictions in that landscape class in an interval of time that precedes the assessment year. In all cases these predictions also allow for the possibility that changes in the future may depend on the state of the receptor impact variable in the reference year 2012, and consequently this is also quantified by conditioning on the predicted hydrological conditions in a reference interval that precedes 2012 (companion submethodology M08 (as listed in Table 1) for receptor impact modelling (Hosack et al., 2018)).
For surface water and groundwater variables in the Gloucester subregion, the reference assessment interval is defined as the 30 years preceding and including 2012 (i.e. 1983 to 2012). For surface water variables in the Gloucester subregion, the short-assessment interval is defined as the 30 years preceding the short-assessment year (i.e. 2013 to 2042), and the long-assessment interval is defined as the 30 years that precede the long-assessment year (i.e. 2073 to 2102). For groundwater, maximum drawdown (metres) and time to maximum drawdown are considered across the full 90-year window: 2013 to 2102.
In BAs, choices about receptor impact variables must balance the project’s time and resource constraints with the objectives of the assessment and the expectations of the community (companion submethodology M10 (as listed in Table 1) for analysing impacts and risks (Henderson et al., 2018)). This choice is guided by selection criteria that acknowledge the potential for complex direct and indirect effects within perturbed ecosystems, and the need to keep the expert elicitation of receptor impact models tractable and achievable (companion submethodology M08 (as listed in Table 1) for receptor impact modelling (Hosack et al., 2018)).
For perennial gravel/cobble streams, the qualitative modelling workshop identified five variables – three flow regimes, groundwater and precipitation – as the hydrological factors that were thought to: (i) be instrumental in maintaining and shaping the ecosystem and/or, (ii) have the potential to change due to coal resource development (Figure 17). All of the ecological components and processes represented in the qualitative model are potential receptor impact variables and all of these, with the exception of upstream recruitment, are predicted to vary as the hydrological factors vary either individually or in combination (Table 8).
Following advice received from participants during (and after) the qualitative modelling workshop, and guided by the availability of experts for the receptor impact modelling workshop, the scope of the BA numerical modelling and the receptor impact variable selection criteria (see Section 2.7.1.2.3), the receptor impact models focused on the following relationships:
- The response of the woody riparian vegetation (WRV) to changes in flow regime 1 (FR1), flow regime 2 (FR2) and depth to groundwater (GW).
- The response of high-flow macroinvertebrates (HF MI) to changes in flow regime 3 (FR3).
- The response of predators (Pred 2) to changes in flow regime 3 (FR3).
The hydrological factors identified by the participants in the qualitative modelling workshops have been interpreted as a set of hydrological response variables. The hydrological response variables are summary statistics that: (i) reflect these hydrological factors and (ii) can be extracted from BA’s numerical surface water and groundwater models during the reference, short- and long-assessment intervals defined previously. The hydrological factors and associated hydrological response variables for the ‘Perennial – gravel/cobble streams’ landscape class are summarised in Table 9. The precise definition of each receptor impact variable, typically a species or group of species represented by a qualitative model node, was determined during the receptor impact modelling workshop and satisfy the generic criteria set out in 2.7.1.2.3.
Using this interpretation of the hydrological response variables, and the receptor impact variable definitions derived during the receptor impact modelling workshop, the relationships identified in the qualitative modelling workshop were formalised into three receptor impact models (Table 10).
Table 9 Summary of the hydrological response variables used in the receptor impact models, together with the signed digraph variables that they correspond to, for the ‘Perennial – gravel/cobble streams’ landscape class in the Gloucester subregion
FR1 = flow regime 1, FR2 = flow regime 2, FR3 = flow regime 3, GW = groundwater, ZQD = zero-flow days (averaged over 30 years)
Table 10 Summary of the three receptor impact models developed for the ‘Perennial – gravel/cobble streams’ landscape class in the Gloucester subregion
Hydrological response variables are defined in Table 9. ZQD = zero-flow days (averaged over 30 years), QBFI = baseflow index, as defined in Table 9
2.7.3.2.3 Receptor impact models
2.7.3.2.3.1 Canopy cover of woody riparian vegetation
Table 11 summarises the elicitation design matrix for the mean percent canopy cover of woody riparian vegetation in the ‘Perennial – gravel/cobble streams’ landscape class. Each row of the design matrix is a separate elicitation scenario defined by a unique combination of hydrological response variable values. Experts are asked to predict the values of the receptor impact variable in the landscape class under each of these scenarios (companion submethodology M08 (as listed in Table 1) for receptor impact modelling (Hosack et al., 2018)). The first six design points – design point identifiers 8, 1, 3, 6, 9 and 7 – address the predicted variability (across the perennial streams in the landscape class during the reference interval) in the overbench (R0.3) and overbank (R3.0) flows that define floods with a return interval of 3.3 events and 0.33 events per year, respectively. The design points 9, 7, 3, and 1 capture the combination of the extremes of each hydrological response variable, whilst design points 6 and 8 capture intermediate points in each hydrological response variable axis. Note that the design point identifiers are simply index variables that identify the row of the elicitation design matrix. They are included here to maintain an auditable path between analysis and reporting.
The first six design points provide for an estimate of the uncertainty in mean percent canopy cover across the landscape class in the reference year 2012 (Yref). The remaining design points represent hydrological scenarios that span the uncertainty in the values of the hydrological response variables in the relevant time period of hydrological history associated with the short- (2042) and long- (2102) assessment years.
Table 11 Elicitation design matrix for the receptor impact model of mean percent canopy cover in the riparian-dependent community in the ‘Perennial – gravel/cobble streams’ landscape class in the Gloucester subregion
The elicitation scenarios allow for the possibility that the response of Y(short) or Y(long) may be conditional on Y(ref). To do this the elicitation scenarios for the short- and long-assessment years take a representative set of values of Y(ref) – calculated from the elicitations conducted for scenarios in the reference year – and use these in the elicitation scenarios for the short- and long-assessment years. All other design points are either default values or values determined by groundwater and surface water modelling. See Table 9 for definitions of hydrological response variables. na = not applicable
Data: Bioregional Assessment Programme (Dataset 5)
Design point identifiers 72 through to 176 (as listed in Table 11) represent combinations of the four hydrological response variables (dmaxRef, tmaxRef, EventsR0.3 and EventsR3.0), together with high and low values of Yref, that respect certain logical constraints; for example, the number of overbank flood events (EventsR3.0) cannot be greater than the number of overbench flood events (EventsR0.3) (companion submethodology M08 (as listed in Table 1) for receptor impact modelling (Hosack et. al., 2018)). The high and low values for Yref were calculated during the receptor impact modelling workshop following the experts’ response to the first six design points, and then automatically included within the design for the elicitations at the subsequent design points.
The receptor impact modelling methodology allows for a very flexible class of statistical models to be fitted to the values of the receptor impact variables elicited from the experts at each of the design points (companion submethodology M08 (as listed in Table 1) for receptor impact modelling (Hosack et al., 2018)). The model fitted to the elicited values of mean percent canopy cover for the ‘Perennial – gravel/cobble streams’ landscape class is summarised in Figure 18 and Table 12. The fitted model takes the form:
|
(1) |
where is an intercept term (a vector of ones),
is a binary indicator variable scored 1 for the case of an assessment in the short- or long-assessment year,
is a binary indicator variable scored 1 for the case of an assessment in the long-assessment year,
is a continuous variable that represent the value of the receptor impact variable in the reference year (Yref, set to zero for the case of an assessment in the reference year), and
are the (continuous or integer) values of the four hydrological response variables (dmaxRef, tmaxRef, EventsR0.3 and EventsR3.0). Note that the modelling framework provides for more complex models, including quadratic value of, and in interactions between, the hydrological response variables but in this instance the simple linear model (Equation 1) was identified as the most parsimonious representation of the experts’ responses.
The model estimation procedure adopts a Bayesian approach. The model coefficients ( are assumed to follow a multivariate normal distribution. The Bayesian estimation procedure finds the parameters of this distribution conditional on the data (the elicited expert opinion) for a number of different models, and then selects the most parsimonious model using a common information criterion (companion submethodology M08 (as listed in Table 1) for receptor impact modelling (Hosack et al., 2018) – in this case a simple linear model. Table 12 summarises the estimated mean and 80% credible interval of the eight model coefficients. Figure 18 shows the resulting model predictions for the (marginal) mean and 80% central credible intervals[1] of the four hydrological response variable.
The model indicates that the experts’ opinion provides strong evidence for Yref having a positive effect on mean percent canopy cover. This suggests that given a set of hydrological response variable values in the future, a site with a higher mean percent canopy cover at the 2012 reference point is more likely to have a higher mean percent canopy cover in the future than a site with a lower mean percent canopy cover value at this time point. This reflects the lag in the response of mean percent canopy cover to changes in hydrological response variables that would be expected of mature trees with long life spans and is relevant to both the short- and long-assessment periods.
The model also indicates that the experts’ opinion provides strong evidence for dmaxRef having a negative effect on mean percent canopy cover. This suggests that mean percent canopy cover will decrease as groundwater drawdown increases due to coal resource development. The model predicts that (holding all other hydrological response variables constant at the mid-point of their elicitation range) the mean of the mean percent canopy cover will drop from just under 50% without any change in groundwater level, to about 35% if the levels decease by 6 m relative to the reference level in 2012. There is, however, considerable uncertainty in these predictions, with an 80% chance that the mean percent canopy cover will lie somewhere between approximately 60% and 20% in the short-assessment period, and somewhere between roughly 62% and 17% in the long-assessment period, with a 6 m drop in groundwater level.
In middle and bottom rows, the uncertainty in mean percent canopy cover in the reference year was integrated out, holding all other hydrological response variables constant at the mid-point of their elicitation range (during risk estimation all hydrological response variables vary simultaneously). Dashed vertical lines show the range of hydrological response variables used in the elicitation. Yrs2tmaxRef is the difference between tmaxRef and the assessment year that is relevant for the prediction (2012, 2042 or 2102). See Table 9 for definitions of hydrological response variables.
The numbers on the y-axis range from 0 to 1 as the receptor impact model was constructed using the proportion for the statistical modelling. They should be interpreted as a percent foliage cover ranging from 0 to 100%.
Data: Bioregional Assessment Programme (Dataset 5)
Table 12 Mean, 10th and 90th percentile of the coefficients of the receptor impact model for mean percent canopy cover in the riparian-dependent community in the ‘Perennial – gravel/cobble streams’ landscape class for the Gloucester subregion
Yref is value of receptor impact variable in the reference year; set to zero if the design point is in the reference assessment year. Future is a binary variable scored 1 if the design point is in a short- or long-assessment year. Long is a binary variable scored 1 if the design point is in the long-assessment year. dmaxRef, EventsR0.3 and EventsR3.0 are as defined in Table 9. Yrs2tmaxRef is the difference between tmaxRef and the assessment year that is relevant for the prediction (2012, 2042 or 2102). These coefficients show, on the transformed scale, the change in the expected value of the receptor impact variable for a unit change in their associated hydrological response variable, ignoring the effect of all other hydrological response variables. This effect cannot be directly interpreted, however, without first fixing all other hydrological response variables to a specific value and second applying the inverse of the model link function.
Data: Bioregional Assessment Programme (Dataset 5)
The fitted model suggests that the evidence for the effect of EventsR0.3 in the experts’ responses is weaker than for the other hydrological response variables discussed above. The 80% credible interval for this hydrological response variable’s coefficient spans zero, whereas the 80% central credible interval of Yref and dmaxRef lie wholly within the positive or negative parts of the real line (Table 12). Nonetheless, if all other hydrological response variables are held at the mid-point of their elicitation range, the model suggests that an increase in the frequency of overbench flows will have a relatively small positive effect on mean percent canopy cover – the predicted slight increase in the average frequency of overbench flood events, from 3.3 (by definition) in the 30 years preceding the reference year, to a maximum average value of 4.6 over the future period, causes the mean percent canopy cover to increase by about 5%. However, the large uncertainty reflected in the 80% credible intervals in Figure 18 does not preclude the small possibility of EventsR0.3 having a negligible effect on mean percent canopy cover.
The summary statistics for the marginal distribution of the model coefficients (Table 12) and the partial regression plots (Figure 18) for all of the other model coefficients indicate that there is insufficient information in the expert-elicited data to determine the effects of the future coefficient, the long coefficient and the coefficients for Yrs2tmaxRef and EventsR3.0. This situation is indicated by relatively large positive (negative) 10th and negative (positive) 90th percentiles in Table 12, parallel slopes in the short and long partial regression plots in Figure 18, and the almost-zero mean (flat slope) of the coefficients for Yrs2tmaxRef and EventsR3.0 in Table 12. With the exception of the last two variables, these results are not surprising as they suggest that the variation in the elicited values of the receptor impact variable can be adequately described by the other hydrological response variables. For Yrs2tmaxRef and EventsR3.0, however, this indicates that either the experts believe that the effect of the rate of groundwater drawdown and overbank floods is very weak compared to that of the other hydrological response variables or that there was insufficient information in the elicited values to adequately quantify the effect of these variables.
2.7.3.2.3.2 Density of Hydropsychidae larvae
Table 13 summarises the elicitation matrix for the density of Hydropsychidae larvae. The first four design points – design point identifiers 8, 1, 6 and 999 – address the predicted variability (across the landscape class in the reference interval) in ZQD, capturing the lowest and highest predicted values together with two intermediate values. These design points provide for an estimate of the uncertainty in Hydropsychidae larval density across the landscape class in the reference year 2012 (Yref).
Design points 16 to 21 inclusive (as listed in Table 13) represent scenarios that span the uncertainty in the predicted values of ZQD in the relevant time period of hydrological history associated with the short- (2042) and long- (2102) assessment years, combined with high and low values of Yref. Again, the high and low values for Yref were calculated during the receptor impact modelling workshop.
The fitted model for Hydropsychidae larval density takes the form:
|
(2) |
where the terms and
are as before and
is the integer value of ZQD. The (marginal) mean and 80% central credible interval of the coefficient for this hydrological response variable are summarised in the partial regression plots in Figure 19, whilst Table 14 summarises the same information for all five model coefficients.
Unlike the previous model, the hydrological response variable in the Hydropsychidae model varies during the reference interval and the future interval. The model indicates that the experts’ elicited information strongly supports the hypothesis that an increase in ZQD will have a negative effect on the density of Hydropsychidae larvae despite the experts being quite uncertain about its average value. The model suggests that larval density can vary substantially across the landscape class from less than 100 per m2 to almost 1000 per m2 under conditions of constant flow (ZQD = 0), holding all other covariates at their mid-values. As the number of zero-flow days (averaged over 30 years) (ZQD, subsequently referred to in this Section as ‘zero-flow days’) increases, however, experts were of the opinion that larval density would drop quite dramatically with values of less than 1 per m2 falling within the 80% credible interval under very intermittent flow conditions (ZQD >200 days) (Figure 19).
There was very little evidence in the elicited data to suggest that this effect would be substantially different in the future assessment years. Again, this is indicated by the almost identical partial regression plots in the reference, short- and long-assessment years (Figure 19), and the relatively large negative 10th and positive 90th percentiles for the long and future coefficients in Table 14. The model does, however, suggest that the experts’ uncertainty increased for predictions in the future assessment years relative to the reference year.
Table 13 Elicitation design matrix for the receptor impact model of the mean density of Hydropsychidae larvae in the ‘Perennial – gravel/cobble streams’ landscape class for the Gloucester subregion
The elicitation scenarios allow for the possibility that the response of Y(short) or Y(long) may be conditional on Y(ref). To do this the elicitation scenarios for the short- and long-assessment years take a representative set of values of Y(ref) – calculated from the elicitations conducted for scenarios in the reference year – and use these in the elicitation scenarios for the short- and long-assessment yearsAll other design points (with identifiers) are either default values or values determined by groundwater and surface water modelling.
na = not applicable, ZQD = zero-flow days (averaged over 30 years)
Data: Bioregional Assessment Programme (Dataset 5)
Another notable difference between this model and the previous model is the estimated values for the Yref coefficient. In the canopy cover model there was strong evidence within the experts’ elicited values that mean percent canopy cover in the reference year had a positive influence on the values in the future assessment years). The best-fitting model in this case, however, is unable to eliminate the possibility that the average density of Hydropsychidae larvae in the reference years has no influence on its density in the future years. This is indicated by the fact that
is close to the 50th percentile of its induced prior (Table 14). This suggestion is consistent with the hypothesis that there is likely to be very little lag in the response of this short-lived species to changes in the hydrological response variables.
In middle and bottom rows, all other hydrological response variables are held constant at the mid-point of their elicitation range (during risk estimation all hydrological response variables vary simultaneously). Dashed vertical lines show range of hydrological response variables used in the elicitation. ZQD = zero-flow days (averaged over 30 years). Note that the apparent extrapolation of the relationship beyond the elicitation range reflects the preliminary hydrological model output for the reference period where there was a high maximum number of zero-flow days. In future periods the [preliminary] hydrological modelling indicated that the maximum number of zero-flow days is around 40. Extrapolation beyond 40 zero-flow days in the future periods is visualised here for comparison with the reference period prediction. (Note that the apparent extrapolation of the relationship beyond the elicitation range reflects the preliminary hydrological model output for the reference period which showed a high maximum number of zero-flow days.)
Data: Bioregional Assessment Programme (Dataset 5)
Table 14 Mean, 10th and 90th percentile of the coefficients of the receptor impact model for the mean density of Hydropsychidae larvae in the riparian-dependent community in the ‘Perennial – gravel/cobble streams’ landscape class for the Gloucester subregion
Mean |
10th percentile |
90th percentile |
|
---|---|---|---|
(Intercept) |
5.18 |
3.79 |
6.58 |
future1 |
0.279 |
–3.32 |
3.88 |
long1 |
0.263 |
–1.08 |
1.61 |
Yref |
–0.139 |
–0.849 |
0.571 |
ZQD |
–0.0207 |
–0.0347 |
–0.00671 |
Yref is value of receptor impact variable in the reference assessment year; set to zero if case is in the reference assessment year. Long is a binary variable scored 1 if the design point is in the long-assessment year. Future is a binary variable scored 1 if the design point is in a short- or long-assessment year. ZQD = zero-flow days (averaged over 30 years)
Data: Bioregional Assessment Programme (Dataset 5)
2.7.3.2.3.3 Density of eel-tailed catfish (Tandanus tandanus)
The elicitation scenarios for the average density of the eel-tailed catfish (Tandanus tandanus) are summarised in Table 15. This elicitation proved to be similar to that for the Hydropsychidae larvae. Although Yref, QBFI and ZQD were considered in the elicitation design, only ZQD was subsequently determined to be predictive of the density of eel-tailed catfish in the future assessment years (see below).
The elicitation scenarios were again chosen to enable the model to estimate the uncertainty in the average density of the eel-tailed catfish in the reference year 2012 (design point identifiers 8 to 7 inclusive as shown in Table 15). Thereafter into the future assessment years, the design points reflect high, medium and low values of the two hydrological response variables (ZQD and QBFI), in combination with high and low values of average density in 2012.
The best-fitting model for the average density of the eel-tailed catfish takes the form:
|
(3) |
where the terms and
are as before,
is the integer value of ZQD, and
is the continuous value of QBFI. The (marginal) mean and 80% central credible interval of the coefficient for the two hydrological response variables are summarised in the partial regression plots in Figure 20, whilst Table 16 summarises the same information for all six model coefficients.
Table 15 Elicitation design matrix for the receptor impact model of the density of eel-tailed catfish (Tandanus tandanus) in the ‘Perennial – gravel/cobble streams’ landscape class for the Gloucester subregion
The elicitation scenarios allow for the possibility that the response of Y(short) or Y(long) may be conditional on Y(ref). To do this the elicitation scenarios for the short- and long-assessment years take a representative set of values of Y(ref) – calculated from the elicitations conducted for scenarios in the reference year – and use these in the elicitation scenarios for the short- and long-assessment years. All other design points (with identifiers) are either default values or values determined by groundwater and surface water modelling.
na = not applicable, QBFI = baseflow index as described in Table 9, ZQD = zero-flow days (averaged over 30 years)
Data: Bioregional Assessment Programme (Dataset 5)
In this model ZQD again varies in the reference year, and the experts’ elicited values for this assessment year provide some evidence that average density of the catfish will decline as ZQD increases, from about 5 individuals per 600 m2 transect under continuous flow (ZQD = zero), holding all other covariates at their mid-values, decreasing to less than 1 individual in two transects as flow becomes more intermittent (ZQD >80 days). Again, however, there is considerable uncertainty in these predictions, and the 80% credible interval does not preclude the possibility of this variable having no further effect on the average denstity of catfish once ZQD increases beyond days.
There was insufficient information in the experts’ elicited response to exclude the possibility that QBFI has no effect on the average density of eel-tailed catfish. This is indicated by the horizontal partial regression plots for QBFI in Figure 20, and the large negative 10th percentile and large positive 90th percentile, with a mean very close to zero, in Table 16. The best-fitting model also does not exclude the possibility that all other covariates, including Yref, have no effect on the average density of the catfish. This again suggests that the experts’ response indicates that the catfish is sufficiently short-lived (generally less than 8 years) such that the experts did not anticipate any significant lag (>30 years) in its response to declines in ZQD.
In middle and bottom rows, all other hydrological response variables are held constant at the mid-point of their elicitation range (during risk estimation all hydrological response variables vary simultaneously). Dashed vertical lines show range of hydrological response variables used in the elicitation. QBFI = index of baseflow as described in Table 9, ZQD = zero-flow days (averaged over 30 years)
Data: Bioregional Assessment Programme (Dataset 5)
Table 16 Mean, 10th and 90th percentile of the coefficients of the receptor impact model for the mean density of eel-tailed catfish (Tandanus tandanus) in the ‘Perennial – gravel/cobble streams’ landscape class for the Gloucester subregion
Mean |
10th percentile |
90th percentile |
|
---|---|---|---|
(Intercept) |
1.57 |
–0.87 |
4.01 |
future1 |
l0.0203 |
–2.19 |
2.23 |
long1 |
0.0333 |
–2.07 |
2.14 |
Yref |
–0.00764 |
–0.714 |
0.699 |
QBFI |
–0.044 |
–5 |
4.91 |
ZQD |
–0.0305 |
–0.065 |
0.00409 |
Yref is value of receptor impact variable in the reference assessment year; set to zero if case is in the reference assessment year. Long is a binary variable scored 1 if the design point is in the long-assessment year. Future is a binary variable scored 1 if the design point is in a short- or long-assessment year. QBFI is the ratio of total baseflow generation to total streamflow generation, averaged over a 30-year period. ZQD = zero-flow days (averaged over 30 years)
Data: Bioregional Assessment Programme (Dataset 5)
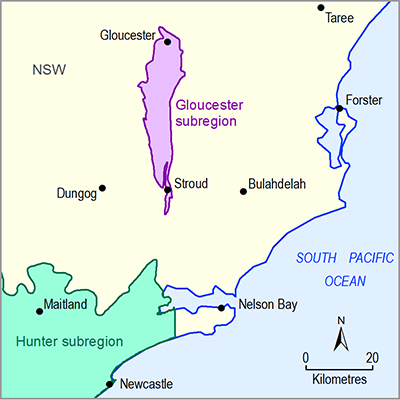